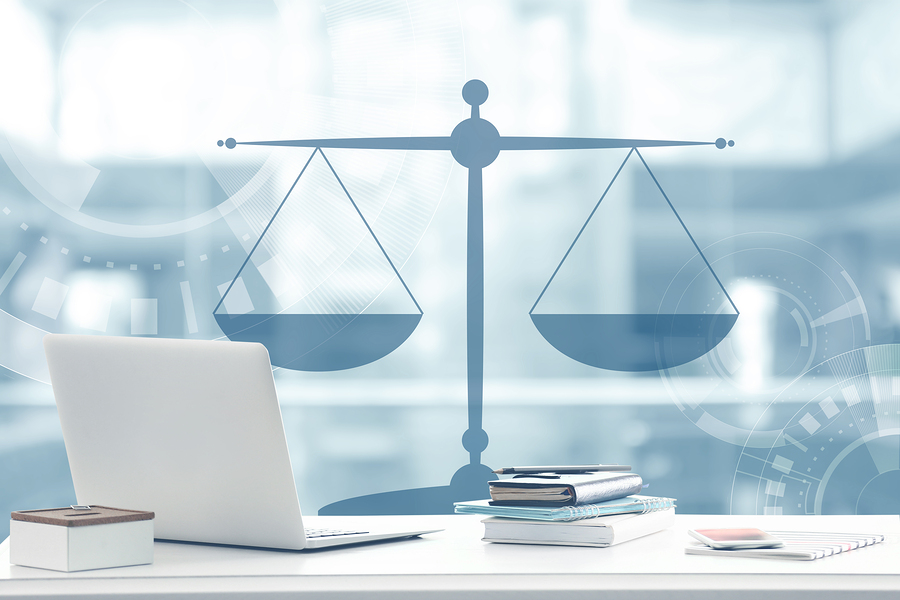
From the Australian government’s new “data-driven profiling” trial for drug testing welfare recipients, to US law enforcement’s use of facial recognition technology and the deployment of proprietary software in sentencing in many US courts … almost by stealth and with remarkably little outcry, technology is transforming the way we are policed, categorized as citizens and, perhaps one day soon, governed.
We are only in the earliest stages of so-called algorithmic regulation – intelligent machines deploying big data, machine learning and artificial intelligence (AI) to regulate human behaviour and enforce laws – but it already has profound implications for the relationship between private citizens and the state.
Furthermore, the rise of such technologies is occurring at precisely the moment when faith in governments across much of the Western world has plummeted to an all-time low. Voters across much of the developed world increasingly perceive establishment politicians and those who surround them to be out-of touch bubble-dwellers and are registering their discontent at the ballot box.
A technical solution
In this volatile political climate, there’s a growing feeling that technology can provide an alternative solution. Advocates of algorithmic regulation claim that many human-created laws and regulations can be better and more immediately applied in real-time by AI than by human agents, given the steadily improving capacity of machines to learn and their ability to sift and interpret an ever-growing flood of (often smartphone-generated) data.
AI advocates also suggest that, based on historical trends and human behaviour, algorithms may soon be able to shape every aspect of our daily lives, from how we conduct ourselves as drivers, to our responsibilities and entitlements as citizens, and the punishments we should receive for not obeying the law. In fact one does not have to look too far into the future to imagine a world in which AI could actually autonomously create legislation, anticipating and preventing societal problems before they arise.
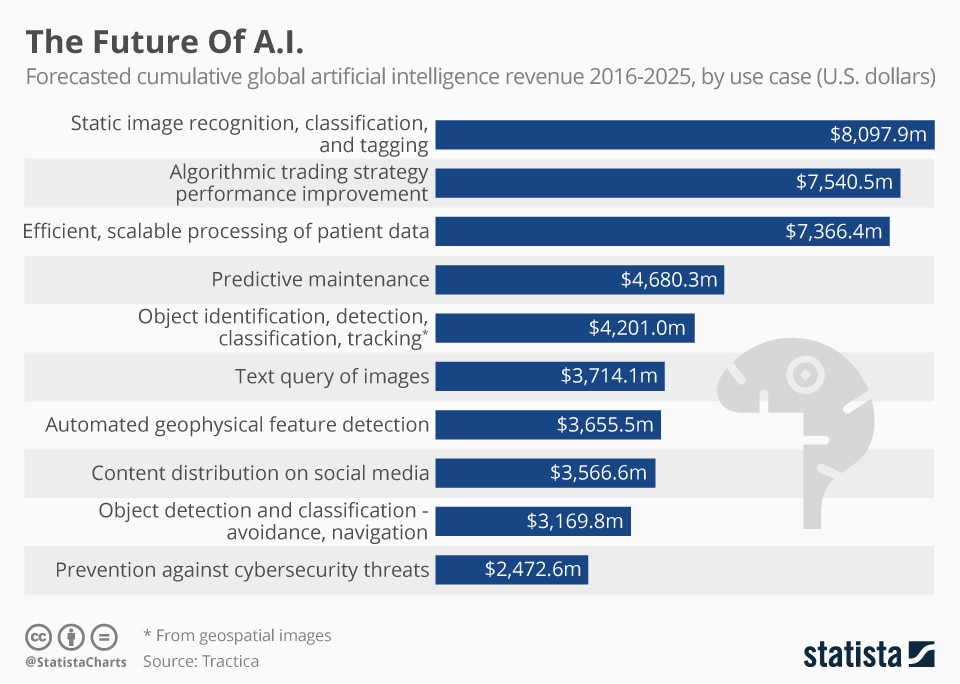
Some may herald this as democracy rebooted. In my view it represents nothing less than a threat to democracy itself – and deep scepticism should prevail. There are five major problems with bringing algorithms into the policy arena:
1) Self-reinforcing bias
What machine learning and AI, in general, excel at (unlike human beings) is analysing millions of data points in real time to identify trends and, based on that, offering up “if this, then that” type conclusions. The inherent problem with that is it carries with it a self-reinforcing bias, because it assumes that what happened in the past will be repeated.
Let’s take the example of crime data. Black and minority neighborhoods with lower incomes are far more likely to be blighted with crime and anti-social behaviour than prosperous white ones. If you then use algorithms to shape laws, what will inevitably happen is that such neighbourhoods will be singled out for intensive police patrols, thereby increasing the odds of stand-offs and arrests.
This, of course, turns perfectly valid concerns about the high crime rate in a particular area into a self-fulfilling prophecy. If you are a kid born in an area targeted in this way, then the chances of escaping your environment grow ever slimmer.
This is already happening, of course. Predictive policing – which has been in use across the US since the early 2010s – has persistently faced accusations of being flawed and prone to deep-rooted racial bias. Whether or not predictive policing can sustainably reduce crime, remains to be proven.
2) Vulnerability to attack
A second and no less important issue around AI-shaped law is security. Virtually all major corporations, government institutions and agencies – including the US Department of Justice – have likely been breached at some point, largely because such organizations tend to lag far behind the hackers when it comes to securing data. It is, to put it mildly, unlikely that governments will be able to protect algorithms from attackers, and as algorithms tend to be “black boxed”, it’s unclear whether we’ll be able to identify if and when an algorithm has even been tampered with.
The recent debate in the US about alleged Russian hacking of the Democratic National Committee, which reportedly aided Donald Trump’s bid to become president, is a case in point. Similarly, owing to the complexity of the code that would need to be written to transfer government and judicial powers to a machine, it is a near certainty, given everything we know about software, that it would be riddled with bugs.
3) Who’s calling the shots?
There is also an issue around conflict of interest. The software used in policing and regulation isn’t developed by governments, of course, but by private corporations, often tech multinationals, who already supply government software and tend to have extremely clear proprietary incentives as well as, frequently, opaque links to government.
Such partnerships also raise questions around the transparency of these algorithms, a major concern given their impact on people’s lives. We live in a world in which government data is increasingly available to the public. This is a public good and I’m a strong supporter of it.
Yet the companies who are benefiting most from this free data surge show double standards: they are fierce advocates of free and open data when governments are the source, but fight tooth and nail to ensure that their own programming and data remains proprietary.
4) Are governments up to it?
Then there’s the issue of governments’ competence on matters digital. The vast majority of politicians in my experience have close to zero understanding of the limits of technology, what it can and cannot do. Politicians’ failure to grasp the fundamentals, let alone the intricacies, of the space means that they cannot adequately regulate the software companies that would be building the software.
If they are incapable of appreciating why backdoors cannot go hand-in-hand with encryption, they will likely be unable to make the cognitive jump to what algorithmic regulation, which has many more layers of complexity, would require.
Equally, the regulations that the British and French governments are putting in place, which give the state ever-expanding access to citizen data, suggest they do not understand the scale of the risk they are creating by building such databases. It is certainly just a matter of time before the next scandal erupts, involving a massive overreach of government.
5) Algorithms don’t do nuance
Meanwhile, arguably reflecting the hubristic attitude in Silicon Valley that there are few if any meaningful problems that tech cannot solve, the final issue with the AI approach to regulation is that there is always an optimal solution to every problem.
Yet fixing seemingly intractable societal issues requires patience, compromise and, above all, arbitration. Take California’s water shortage. It’s a tale of competing demands – the agricultural industry versus the general population; those who argue for consumption to be cut to combat climate change, versus others who say global warming is not an existential threat. Can an algorithm ever truly arbitrate between these parties? On a macro level, is it capable of deciding who should carry the greatest burden regarding climate change: developed countries, who caused the problem in the first place, or developing countries who say it’s their time to modernize now, which will require them to continue to be energy inefficient?
My point here is that algorithms, while comfortable with black and white, are not good at coping with shifting shades of gray, with nuance and trade-offs; at weighing philosophical values and extracting hard-won concessions. While we could potentially build algorithms that implement and manage a certain kind of society, we would surely first need to agree what sort of society we want.
And then what happens when that society undergoes periodic (rapid or gradual) fundamental change? Imagine, for instance, the algorithm that would have been built when slavery was rife, being gay was unacceptable and women didn’t have the right to vote. Which is why, of course, we elect governments to base decisions not on historical trends but on visions which the majority of voters buy into, often honed with compromise.
Much of what civil societies have to do is establish an ever-evolving consensus about how we want our lives to be. And that’s not something we can outsource completely to an intelligent machine.
Setting some ground rules
All the problems notwithstanding, there’s little doubt that AI-powered government of some kind will happen. So, how can we avoid it becoming the stuff of bad science fiction?
To begin with, we should leverage AI to explore positive alternatives instead of just applying it to support traditional solutions to society’s perceived problems. Rather than simply finding and sending criminals to jail faster in order to protect the public, how about using AI to figure out the effectiveness of other potential solutions? Offering young adult literacy, numeracy and other skills might well represent a far superior and more cost-effective solution to crime than more aggressive law enforcement.
Moreover, AI should always be used at a population level, rather than at the individual level, in order to avoid stigmatizing people on the basis of their history, their genes and where they live. The same goes for the more subtle, yet even more pervasive data-driven targeting by prospective employers, health insurers, credit card companies and mortgage providers. While the commercial imperative for AI-powered categorization is clear, when it targets individuals it amounts to profiling with the inevitable consequence that entire sections of society are locked out of opportunity.
To be sure, not all companies use data against their customers. When a 2015 Harvard Business School study, and subsequent review by Airbnb, uncovered routine bias against black and ethnic minority renters using the home-sharing platform, Airbnb executives took steps to clamp down on the problem. But Airbnb could have avoided the need for the study and its review altogether, because a really smart application of AI algorithms to the platform’s data could have picked up the discrimination much earlier and perhaps also have suggested ways of preventing it. This approach would exploit technology to support better decision-making humans, rather than displace humans as decision-makers.
To realize the potential of this approach in the public sector, governments need to devise a methodology that starts with a debate about what the desired outcome would be from the deployment of algorithms, so that we can understand and agree exactly what we want to measure the performance of the algorithms against.
Secondly – and politicians would need to get up to speed here – there would need to be a real-time and constant flow of data on algorithm performance for each case in which they are used, so that algorithms can continually adapt to reflect changing circumstances and needs.
Thirdly, any proposed regulation or legislation that is informed by the application of AI should be rigorously tested against a traditional human approach before being passed into law.
Finally, any for-profit company that uses public sector data to strengthen or improve its own algorithm should either share future profits with the government or agree an arrangement whereby said algorithm will at first be leased and, eventually, owned by the government.
Make no mistake, algorithmic regulation is on its way. But AI’s wider introduction into government needs to be carefully managed to ensure that it’s harnessed for the right reasons – for society’s betterment – in the right way. The alternative risks a chaos of unintended consequences and, ultimately, perhaps democracy itself.